Cells: The Bedrock of Biology
Cells are the fundamental unit of biology and have long been studied at both the functional level with markers and tools such as Fluorescence-activated Cell Sorting (FACS) and Flow Cytometry, and at the molecular level with genetic, genomic, transcriptomic, and proteomic analyses. More recently various initiatives such as Tabula Sapiens1 and the Human Cell Atlas2 have combined single-cell and spatial resolution to further characterize cells. And while significant technical advances have ushered in a new era of multi-omic discoveries that combine these analytes and methods, it’s important not to lose sight of the rich data that cell morphology has to offer.
Morphology, as the study of size, shape and structure of cells, is an important modality to predict disease state, stage, and to characterize cells. Researchers have used interpretable qualitative and quantitative measurements of morphology, or morphometrics, to predict distinct tumorigenic and metastatic potentials3, to do phenotypic analyses in diseases like sickle cell4, and to do functional screening in drug discovery5.
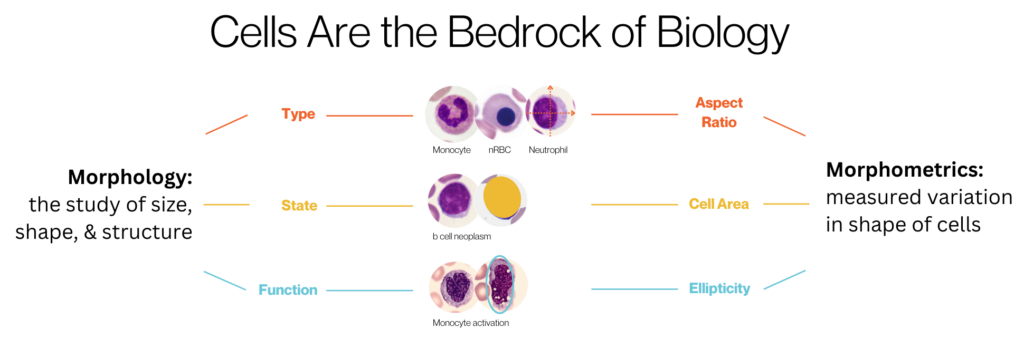
At the core of quantifying morphology for these applications are morphometrics and human interpretation, but cell morphology is as diverse as individual humans. Artificial intelligence (AI), and specifically deep learning using neural networks, is powerful for leveraging images of cells to interpret their unique morphology created from their complex molecular networks6. Deep learning is being applied to predicting biological behavior of cancer7 and automating the segmentation of cells in tissue images8, providing deeper insights into cell phenotypes beyond traditional interpretable morphometrics.
Deep Learning for Deep Insights
Deepcell is pioneering an innovative area of single-cell biology analysis focused on multidimensional readouts of cell morphology without the requirement of cellular markers. We combine human expertise, morphometrics, and additional AI-defined metrics to understand cells more effectively than any one of those three alone. With the Deepcell platform, captured brightfield images of cells flowing inside a microfluidic channel are analyzed by deep learning models in real-time, and cells of interest can be routed for collection for downstream molecular or other analyses. In addition, the Deepcell Cloud allows users to explore the data through interactive UMAPs, population analytics, and image visualizations. Using these features, investigators can define and save cell populations of interest, and reproducibly use them for subsequent analysis or retrieval. In fact, as part of our Technology Access Program (TAP), scientific collaborators are already applying this method to drugged lymphoblasts and copy number profiling of enriched tumor cell fractions with promising results.
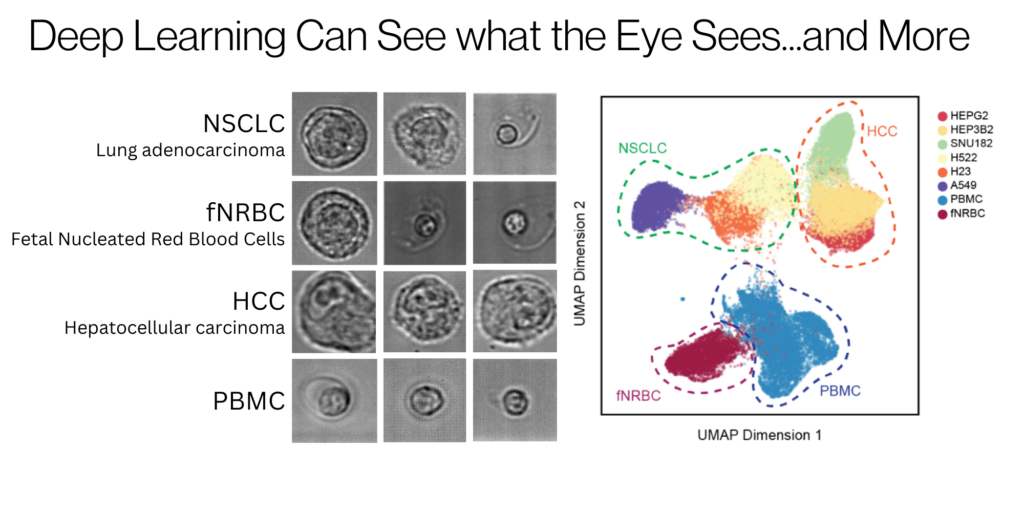
An AI-Powered Platform for Discovery and Refinement
This single-cell morphology characterization technology has many applications across biology, from basic to translational research. With the ability to deeply characterize cells, atlasing initiatives can benefit from including high-dimensional morphology as an added data type. In addition, better understanding the heterogeneity of cells in cancer and other diseases offer a promising path to more targeted and effective therapeutics. Lastly, morphological readouts can provide a straightforward method for functional screens of molecular and chemical perturbations. So, it begs the question: what will you discover with high-dimensional morphology data?
References:
- Tabula Sapiens Consortium. (2019). Human transcriptome reference at single cell resolution. https://tabula-sapiens-portal.ds.czbiohub.org
- Human Cell Atlas. (2016). To create comprehensive reference maps of all human cells. https://www.humancellatlas.org
- Wu, P., et al., (2020). Single-cell morphology encodes metastatic potential. Science Advances, 6(4). https://www.science.org/doi/full/10.1126/sciadv.aaw6938
- Ozpolat, T., et al., (2022). Phenotypic analysis of erythrocytes in sickle cell disease using imaging flow cytometry. Journal of Quantitative Cell Science, 101(5), 448-457. https://onlinelibrary.wiley.com/doi/abs/10.1002/cyto.a.24536
- Kusumoto, D., et al., (2021). Anti-senescent drug screening by deep learning-based morphology senescence scoring. Nature Communications, 12, Article 257. https://www.nature.com/articles/s41467-020-20213-0
- Pratapa, A., et al., (2021). Image-based cell phenotyping with deep learning. Current Opinion in Chemical Biology, 65, 9-17. https://www.sciencedirect.com/science/article/abs/pii/S1367593121000478?via%3Dihub
- Da, Q., et al., (2022). Quantifying the cell morphology and predicting biological behavior of signet ring cell carcinoma using deep learning. Scientific Reports, 12, Article 183. https://www.nature.com/articles/s41598-021-03984-4
- Greenwald, N., et al., (2021). Whole-cell segmentation of tissue images with human-level performance using large-scale data annotation and deep learning. Nature Biotechnology, 40, 555-565. https://www.nature.com/articles/s41587-021-01094-0
—
Intrigued by what you read? Join our email list to stay informed about the applications of high-dimensional morphology data.
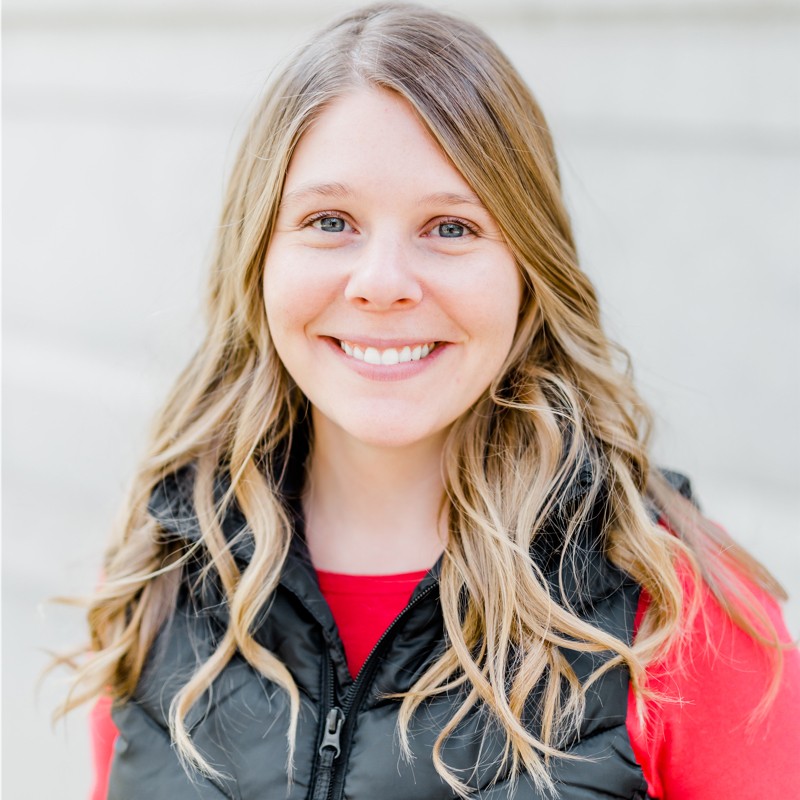
Michelle Balakrishnan
Sr. Product Marketing Manager - Deepcell