Albert Einstein once famously said: “Imagination is more important than knowledge. For knowledge is limited, whereas imagination embraces the entire world.” I believe we need the power of imagination more than ever in the field of biology.
Our most cutting-edge life science tools, drawing from a diverse array of disciplines, are not only engineering projects with complex inner workings, but also often come with interfaces that aren’t designed for use by the masses. Meanwhile, the wet lab itself is subject to confounders that are hard to control. Biological samples are sensitive and slight changes in the physical environment or the operating procedure can leave unwanted traces in the data.
Enter generative AI (GenAI), a sphere of innovation that we at Deepcell are exploring. The end goal? Not merely to imagine, but to vividly conceptualize, via GenAI, cells under conditions that are considered painstakingly difficult or, in some instances, seemingly impossible to produce using wet lab methods. Imagine being able to ask and answer a question such as “what would my cells look like after 5 days of drug treatment?” without the need for actually securing the drugs, adhering to a strict treatment protocol, and manipulating the cells through a physical system for evaluation. These are the types of questions we are hoping to answer with GenAI.
Our journey begins with a focal point: cell morphology. As part of our core product, we trained a foundation model of human cells. This foundation model has seen a large collection of single cell images of many types and states and is able to represent cell morphology in an unsupervised way. Our foundation model will also serve as the bedrock fora groundbreaking generative AI tool, allowing us to envision cells by utilizing any conceivable representation present within the latent space of our model. This latent space is a multi-dimensional space where the model encodes various features and representations allowing for versatile and creative exploration.
The ability to visualize any point—real or hypothetical—within this latent space as a cell will provide insights into the dynamic shifts in morphology across different phenotypic or genotypic characteristics.
Consider, for instance, the Epithelial-Mesenchymal Transition (EMT), a pivotal biological process. With GenAI, we can follow the journey of an “imagined” epithelial cell undergoing EMT, unraveling the intricate metamorphosis. The cell images are generated by a stable diffusion model trained using our foundation model. The colors in the visualization below correspond to different pixel ranges, providing a nuanced perspective on the predicted brightfield image of the cell.
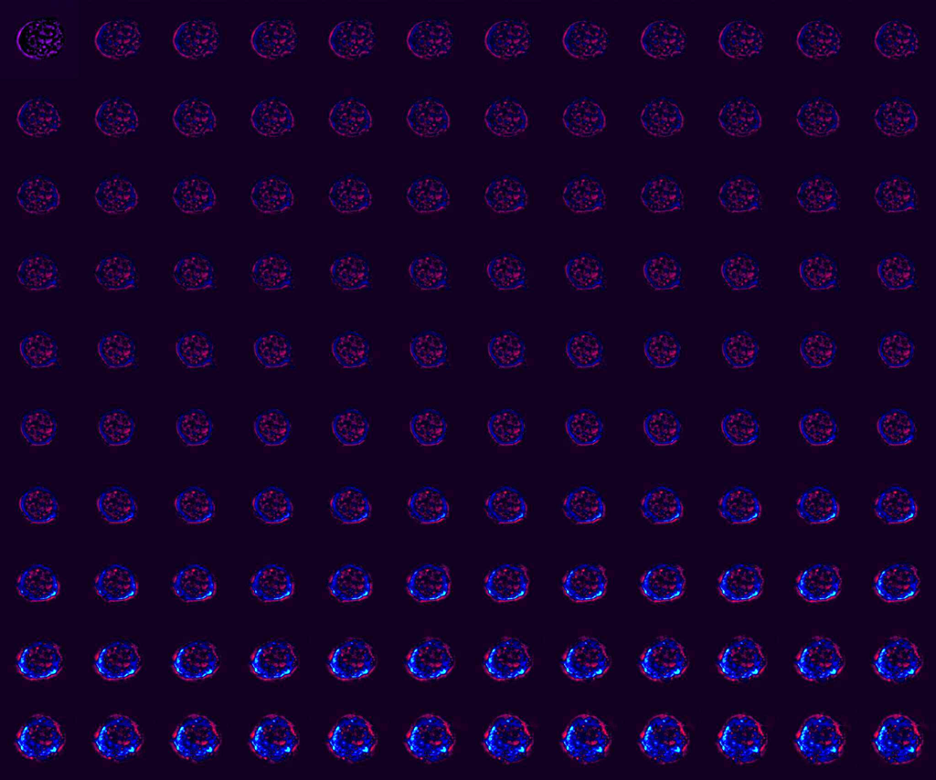
Figure 1: Step-by-step transition of an “imagined” epithelial melanocyte to mesenchymal state generated by a diffusion model trained on top of Deepcell’s foundation model.
Beyond the morphology output lies the capacity to explore and interpret each dimension of the model output, which is currently more than 100, affording us an invaluable understanding of the inner workings of classification algorithms reliant on this foundation model.
However, morphology is merely the prologue to what lies ahead. This trajectory extends to the development of a multimodal GenAI model capable of suggesting nuanced many-to-many mappings between different modalities, such as genomics and spatial transcriptomics. This GenAI approach to cell biology opens up the world of hypothesis generation—hypotheses that are not just exploratory but hold tangible value in scientific inquiry.
We stand at the precipice of a transformative era, where GenAI could revolutionize the field of biology, accelerating discoveries in ways never “imagined” before. This new generation of AI is poised to redefine not just the future of technology but the very essence of scientific exploration itself.
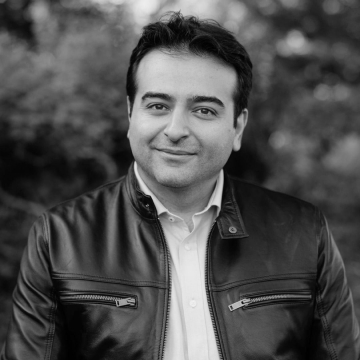
Mahyar Salek
President, Chief Technology Officer, Co-Founder